The Power of AI: How Stable Diffusion and Styly Reshaping the Creativity
The Power of AI: How Stable Diffusion and Styly Reshaping the Creativity
June 18, 2024
Table of Contents
Introduction
We live in a world where artists are losing their jobs, because you can generate whatever piece of art you want with a simple text prompt, within a few seconds, that looks incredibly good. More than that, you can generate an image of anything, even things that don't exist in real life, just by using the right descriptions. This is the power of Stable Diffusion, a state-of-the-art image generation model that is reshaping the creative landscape.
Table of Contents
Introduction
We live in a world where artists are losing their jobs, because you can generate whatever piece of art you want with a simple text prompt, within a few seconds, that looks incredibly good. More than that, you can generate an image of anything, even things that don't exist in real life, just by using the right descriptions. This is the power of Stable Diffusion, a state-of-the-art image generation model that is reshaping the creative landscape.
Table of Contents
Introduction
We live in a world where artists are losing their jobs, because you can generate whatever piece of art you want with a simple text prompt, within a few seconds, that looks incredibly good. More than that, you can generate an image of anything, even things that don't exist in real life, just by using the right descriptions. This is the power of Stable Diffusion, a state-of-the-art image generation model that is reshaping the creative landscape.
Table of Contents
Introduction
We live in a world where artists are losing their jobs, because you can generate whatever piece of art you want with a simple text prompt, within a few seconds, that looks incredibly good. More than that, you can generate an image of anything, even things that don't exist in real life, just by using the right descriptions. This is the power of Stable Diffusion, a state-of-the-art image generation model that is reshaping the creative landscape.
The Convolutional Layer
At the heart of Stable Diffusion lies the convolutional layer, a special type of neural network layer that is particularly well-suited for processing images. Convolutional layers work by applying a small, 2D grid of numbers (called a kernel) to the input image, where each output pixel is determined by the surrounding input pixels and the corresponding kernel values. This allows the network to extract important features from the image, such as edges and textures, without having to worry about the massive number of parameters that would be required in a fully connected layer.
The Evolution of Computer Vision
The development of convolutional networks has led to a rapid advancement in computer vision, with tasks ranging from simple image classification to complex semantic segmentation and instance segmentation. Stable Diffusion builds upon these advances, using a technique called semantic segmentation to understand the contents of an image before generating a new one.
The UNET Architecture
The UNET architecture, a type of convolutional network that is particularly well-suited for image segmentation tasks, plays a key role in Stable Diffusion. UNET works by first downsampling the input image to extract features, and then upsampling the features back to the original resolution, using skip connections to preserve detailed information.
Denoising with Diffusion Models
Stable Diffusion takes a unique approach to image generation, using a technique called diffusion models. Instead of generating images from scratch, diffusion models start with a noisy image and gradually remove the noise, guided by a neural network that has been trained to predict the noise at each step. This process is highly efficient and allows Stable Diffusion to generate high-quality images at a much faster rate than traditional generative models.
The Convolutional Layer
At the heart of Stable Diffusion lies the convolutional layer, a special type of neural network layer that is particularly well-suited for processing images. Convolutional layers work by applying a small, 2D grid of numbers (called a kernel) to the input image, where each output pixel is determined by the surrounding input pixels and the corresponding kernel values. This allows the network to extract important features from the image, such as edges and textures, without having to worry about the massive number of parameters that would be required in a fully connected layer.
The Evolution of Computer Vision
The development of convolutional networks has led to a rapid advancement in computer vision, with tasks ranging from simple image classification to complex semantic segmentation and instance segmentation. Stable Diffusion builds upon these advances, using a technique called semantic segmentation to understand the contents of an image before generating a new one.
The UNET Architecture
The UNET architecture, a type of convolutional network that is particularly well-suited for image segmentation tasks, plays a key role in Stable Diffusion. UNET works by first downsampling the input image to extract features, and then upsampling the features back to the original resolution, using skip connections to preserve detailed information.
Denoising with Diffusion Models
Stable Diffusion takes a unique approach to image generation, using a technique called diffusion models. Instead of generating images from scratch, diffusion models start with a noisy image and gradually remove the noise, guided by a neural network that has been trained to predict the noise at each step. This process is highly efficient and allows Stable Diffusion to generate high-quality images at a much faster rate than traditional generative models.
The Convolutional Layer
At the heart of Stable Diffusion lies the convolutional layer, a special type of neural network layer that is particularly well-suited for processing images. Convolutional layers work by applying a small, 2D grid of numbers (called a kernel) to the input image, where each output pixel is determined by the surrounding input pixels and the corresponding kernel values. This allows the network to extract important features from the image, such as edges and textures, without having to worry about the massive number of parameters that would be required in a fully connected layer.
The Evolution of Computer Vision
The development of convolutional networks has led to a rapid advancement in computer vision, with tasks ranging from simple image classification to complex semantic segmentation and instance segmentation. Stable Diffusion builds upon these advances, using a technique called semantic segmentation to understand the contents of an image before generating a new one.
The UNET Architecture
The UNET architecture, a type of convolutional network that is particularly well-suited for image segmentation tasks, plays a key role in Stable Diffusion. UNET works by first downsampling the input image to extract features, and then upsampling the features back to the original resolution, using skip connections to preserve detailed information.
Denoising with Diffusion Models
Stable Diffusion takes a unique approach to image generation, using a technique called diffusion models. Instead of generating images from scratch, diffusion models start with a noisy image and gradually remove the noise, guided by a neural network that has been trained to predict the noise at each step. This process is highly efficient and allows Stable Diffusion to generate high-quality images at a much faster rate than traditional generative models.




Stable Diffusion and the Latent Space
To further improve the efficiency of Stable Diffusion, the model encodes the input images and text into a compact latent space, reducing the amount of data that needs to be processed. This latent space representation is then used to guide the diffusion process, allowing the model to generate high-quality images from much less data.
The Power of Word Embeddings
Stable Diffusion's ability to generate images from text prompts is made possible by the use of word embeddings, a technique that represents words as numerical vectors in a high-dimensional space. These word embeddings capture the semantic relationships between words, allowing the model to understand the meaning and context of the text prompts.
Self-Attention and Cross-Attention
Stable Diffusion uses two types of attention layers to integrate the information from the text prompts and the image data: self-attention, which operates on the text or image data alone, and cross-attention, which combines the text and image information. These attention mechanisms allow the model to identify the most relevant features in both the text and the image, enabling it to generate highly relevant and coherent images.
Conclusion
Stable Diffusion is a groundbreaking achievement in the field of AI-powered image generation, leveraging a deep understanding of convolutional networks, diffusion models, and word embeddings to create high-quality images from text prompts. As this technology continues to evolve, it holds immense potential to transform the way we create, imagine, and visualize our ideas.
For interior designers, architects, and creative professionals, tools like Styly.io offer an exciting opportunity to harness the power of Stable Diffusion and other AI-driven image-generation capabilities. With Styly, users can seamlessly integrate these advanced technologies into their design workflows, generating photorealistic visualizations, experimenting with different concepts, and bringing their visions to life like never before.
By tapping into the boundless creative potential of Stable Diffusion, Styly empowers designers to push the boundaries of what's possible, turning even the most imaginative ideas into tangible realities. Whether you're reimagining a living room idea, designing a cutting-edge office space, or envisioning an entirely new architectural masterpiece, Styly's AI-powered tools can help you bring your design dreams to life.
As the world of AI-generated imagery continues to evolve, Styly remains at the forefront, offering users a transformative platform to explore, experiment, and elevate their design aspirations. So why settle for anything less? Unlock the full potential of Stable Diffusion and other advanced AI technologies with Styly, and let your creativity soar to new heights.
Stable Diffusion and the Latent Space
To further improve the efficiency of Stable Diffusion, the model encodes the input images and text into a compact latent space, reducing the amount of data that needs to be processed. This latent space representation is then used to guide the diffusion process, allowing the model to generate high-quality images from much less data.
The Power of Word Embeddings
Stable Diffusion's ability to generate images from text prompts is made possible by the use of word embeddings, a technique that represents words as numerical vectors in a high-dimensional space. These word embeddings capture the semantic relationships between words, allowing the model to understand the meaning and context of the text prompts.
Self-Attention and Cross-Attention
Stable Diffusion uses two types of attention layers to integrate the information from the text prompts and the image data: self-attention, which operates on the text or image data alone, and cross-attention, which combines the text and image information. These attention mechanisms allow the model to identify the most relevant features in both the text and the image, enabling it to generate highly relevant and coherent images.
Conclusion
Stable Diffusion is a groundbreaking achievement in the field of AI-powered image generation, leveraging a deep understanding of convolutional networks, diffusion models, and word embeddings to create high-quality images from text prompts. As this technology continues to evolve, it holds immense potential to transform the way we create, imagine, and visualize our ideas.
For interior designers, architects, and creative professionals, tools like Styly.io offer an exciting opportunity to harness the power of Stable Diffusion and other AI-driven image-generation capabilities. With Styly, users can seamlessly integrate these advanced technologies into their design workflows, generating photorealistic visualizations, experimenting with different concepts, and bringing their visions to life like never before.
By tapping into the boundless creative potential of Stable Diffusion, Styly empowers designers to push the boundaries of what's possible, turning even the most imaginative ideas into tangible realities. Whether you're reimagining a living room idea, designing a cutting-edge office space, or envisioning an entirely new architectural masterpiece, Styly's AI-powered tools can help you bring your design dreams to life.
As the world of AI-generated imagery continues to evolve, Styly remains at the forefront, offering users a transformative platform to explore, experiment, and elevate their design aspirations. So why settle for anything less? Unlock the full potential of Stable Diffusion and other advanced AI technologies with Styly, and let your creativity soar to new heights.
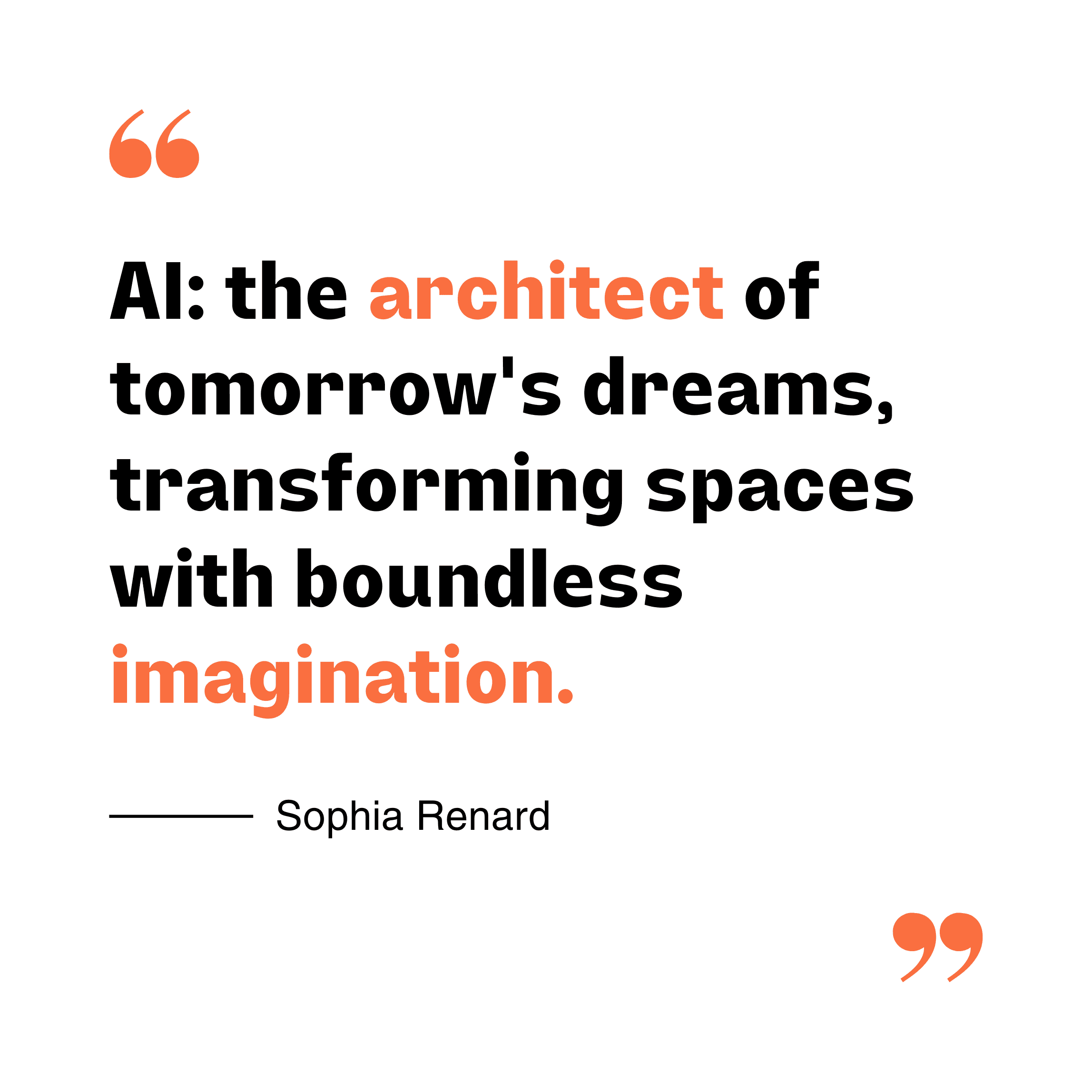